Welcome to Digital Tea with Mr. E. where we will discuss what’s brewing in the world of educational theory. This blog is the sixth of an eight episode series where we will explore T-CAP: A Student Learning Model, and its fit for the modern digital classroom. As I write this blog in the woods surrounding my property on a 54° F late autumn afternoon, I am sipping a cup of Twinings Herbal Orange and Cinnamon Spice tea in a Camp Tuckahoe mug. In Perry County hunting season has begun. Numerous students throughout the day shared their plans to go hunting after school today. So in true Perry County spirit I also went outside after school to hunt deer. As you can see I got the shot: a Tuckahoe 4-point buck with my trusty Nikon Z-7 24mm camera and lens. Then unable to leave the perfect environment, I sat on a tree stump to enjoy the beautiful fall weather and experience the aromatic seasonal tea among the trees of gold.
In this sixth episode of exploring the T-CAP student learning model, I have the results to share for the inter-rater reliability study. Reliability is defined as the instrument’s ability to produce consistent results (Li, 2016). Inter-rater reliability is a research method designed to assess the degree at which multiple raters agree with their assessments of student artifacts. In this case the T-CAP assessment instrument version 2 was used by the six member rater team to assess 6 Physics quests. Version 2 of the T-CAP instrument was made possible through the experience obtained from the T-CAP validity study and the comments provided by the validity team.
The inter-rater reliability team first met on October 22nd during professional development time at school. The reliability team consisted of six teachers in the following disciplines: two agriculture science, one chemistry, one physics, one elementary science, and one English language arts. I passed out hard copy T-CAP assessment rubrics and scoring sheets to each participant. Each reliability rater was also provided with a shared Google Folder containing 6 student quest pdf files, digital copies of the rubric/score sheet, and a content comment document. I added the content comment document to provide a resource of my content comments on the student quests so that the five non physics teachers would have insight regarding the accuracy of the physics equations and mathematics in the quests if needed. One of the raters expressed that this document reduced her anxiety of accurately assessing the physics content learning. The other raters indicated there was no need to use the comment document. I then discussed the intent of finding evidence in the student quests to determine the T-CAP dimension scores. We also discussed how to determine the T-CAP placement based on the dimension scores. Lastly, we agreed upon November 1st as the due date for reliability rater evaluations.
Over the next week and half the reliability rater evaluations trickled in. On November 1st the last evaluations came in on time. With great appreciation I thanked each member of the reliability rater team for their efforts and contributions to the research based evolution of T-CAP. Now it was time to go home and scrap any Friday night social time. I was compelled to crunch the data and learn just how reliable or not the T-CAP assessment instrument is.
In the graphic below I calculated the Cronbach's Alpha for four scenarios: 1) Quest placement on the T-CAP model, 2) isolated Content Learning, 3) isolated Technology Learning, and 4) Isolated Artifact production. I also calculated the standard deviation for the three isolated data sets. The Cronbach Alpha analysis allowed for the assessment of internal consistency of the T-CAP assessment tool and individual dimensions (Zaionitz, 2015). Through standard deviation analysis, I was also able to get an indication of how far the rater responses deviated from the mean values and get a second statistical opinion on the inter-rater reliability of T-CAP dimensions, (Data Star, 2019).
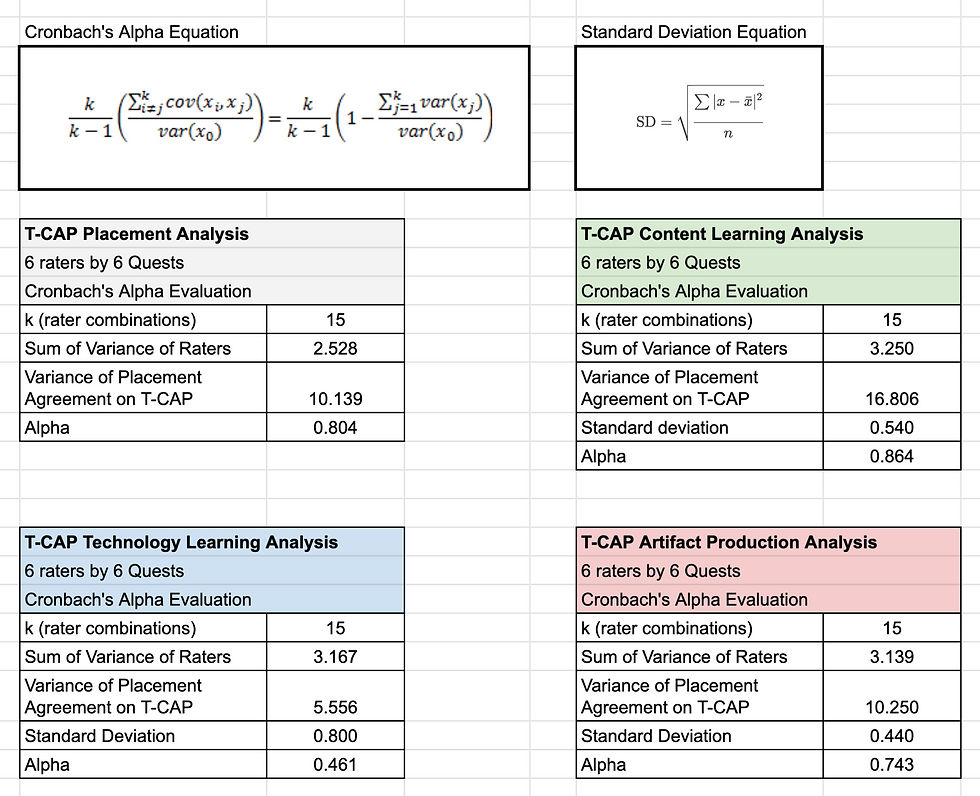
Charles Zaionitz describes Cronbach’s Alpha results of at least 0.7 as good for research accepted internal reliability, and 0.8 as very good for demonstrating internal reliability. Zaionitz also notes that Cronbach’s Alpha results in excess of 0.95 on the 1.0 max scale begin to indicate that there is not enough reasonable variance for authentic research. As you can see from the graphic above the T-CAP Placement Analysis yielded a Cronbach Alpha of 0.804 placing it in the very good category for internal reliability. This result is exciting as it also deems the T-CAP assessment tool is fit for research publication.
I also found the isolated dimension analysis of content learning, technology learning and artifact production to be very valuable. Looking at the Cronbach Alpha results content learning shows very good internal reliability at 0.864, and artifact production shows good internal reliability at 0.743. However, the technology learning dimension only achieved a Cronbach Alpha of 0.461.
This indicates that technology learning dimension of the T-CAP rubric is too ambiguous as internal reliability is only 65% of the amount needed to demonstrate good internal reliability. The technology learning result is further confirmed by the standard deviation calculations that show 0.800 variance on 1-5 Likert scale of novice to distinguished. The content learning and artifact production standard deviations are respectively 0.440 and 0.540, indicating significantly less variance than technology learning. I do not view this result as a failure. The 0.800 technology learning variance falls just short of the 0.75 or less standard I held for the assessment items in the validity study. However, this data does indicate the need for further research and deep thought to improve the clarity of the technology learning dimension on the T-CAP assessment tool.
Some of the raters provided feedback regarding their experience rating the quests. One rater indicated that the T-CAP instrument doesn’t account for when a student’s content learning goals and demonstrated effectiveness do not match. I see where that can make evaluating between striving and proficient a difficult decision. Another comment noted that instrument calls for correctly formatted citations, but not every project involves research and citations. This fact caused difficulties in rating for at least two raters. Another rater indicated that the technology domain was difficult to assess. This comment is further evidence for the need to revise the technology learning dimension.
Episode VII of this eight part series will journal my internship experiences in the time frame from November 4th to 18th. In that time frame I will be writing the analysis and conclusion sections to my T-CAP research paper. I also plan to revise the technology learning dimension of the T-CAP assessment tool. In addition to further research, I hope to gain collaborative support from the reliability team to revise the technology learning dimension. Also, the seventh post will feature a new brew of tea in a special mug. I look forward to sharing the progression of my T-CAP research throughout this internship. Feel free to leave comments below. You may also contact me privately at jeverett@greenwoodsd.org.
References
Data Star, Inc. (2019, November 2). How to Interpret Standard Deviation and Standard Error in Survey Research. Retrieved from http://www.surveystar.com/startips/std_dev.pdf.
Li, Yue. (2016, November 28). How to determine the validity and reliability of an instrument. Retrieved from https://blogs.miamioh.edu/discovery-center/2016/11/ how-to-determine-the-validity-and-reliability-of-an-instrument/.
Zaionitz, Charles. (2015, April 3). Real statistics using excel: Chronbach’s alpha. Retrived from http://www.real-statistics.com/reliability/cronbachs-alpha/comment-page-1/
Well, it is official now. You have a very strong instrument on your hands here. T-CAP can be measured. As we have discussed, the aims for your research internship are very unique. You are trying to prove that this model exists. The fact that it is possible to measure it makes that point. The other goal is to find utility for it. This is more theory-based. I look forward to learning your thoughts on this in your conclusion. Congrats! On a side note, I trust no deer were harmed in the creation of T-CAP!